this is called the distributivity of implication over disjunction in classical propositional logic

YouTube Video
Click to view this content.
The winner outran the fastest horse by over 10 minutes in the 22-mile race held in mid Wales.


YouTube Video
Click to view this content.
A curated list of awesome mathematics resources. Contribute to rossant/awesome-math development by creating an account on GitHub.

some links are broken but otherwise good. Post your open source math textbooks here
As Oklahoma pushes ahead with plans for the first ever taxpayer-funded Catholic public charter school, some say other religions should be included.
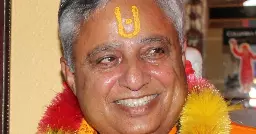
cross-posted from: https://lemmy.sdf.org/post/43170
> >
Prompting is the primary way to utilize the multitask capabilities of language models (LMs), but prompts occupy valuable space in the input context window, and repeatedly encoding the same prompt is computationally inefficient. Finetuning and distillation methods allow for specialization of LMs with...

cross-posted from: https://lemmy.sdf.org/post/36227
> Abstract: "Prompting is now the primary way to utilize the multitask capabilities of language models (LMs), but prompts occupy valuable space in the input context window, and re-encoding the same prompt is computationally inefficient. Finetuning and distillation methods allow for specialization of LMs without prompting, but require retraining the model for each task. To avoid this trade-off entirely, we present gisting, which trains an LM to compress prompts into smaller sets of "gist" tokens which can be reused for compute efficiency. Gist models can be easily trained as part of instruction finetuning via a restricted attention mask that encourages prompt compression. On decoder (LLaMA-7B) and encoder-decoder (FLAN-T5-XXL) LMs, gisting enables up to 26x compression of prompts, resulting in up to 40% FLOPs reductions, 4.2% wall time speedups, storage savings, and minimal loss in output quality. "